by Jesmin Akther | Aug 30, 2021 | Artificial Intelligence
Reinforcement Learning
This type of learning is used to reinforce or strengthen t
he network based on critic information. That is, a network being trained under reinforcement learning, receives some feedback from the environment. However, the feedback is evaluative and not instructive as in the case of supervised learning. Based on this feedback, the network performs the adjustments of the weights to obtain better critic information in future. This learning process is similar to supervised learning but we might have very less information. The following figure gives the block diagram of reinforcement learning.
Building Blocks: Environment and Agent
Environment and Agent are main building blocks of reinforcement learning in AI. This section discusses them in detail −
Agent
An agent is anything that can perceive its environment through sensors and acts upon that environment through effectors.
- A human agent has sensory organs such as eyes, ears, nose, tongue and skin parallel to the sensors, and other organs such as hands, legs, mouth, for effectors.
- A robotic agent replaces cameras and infrared range finders for the sensors, and various motors and actuators for effectors.
- A software agent has encoded bit strings as its programs and actions.
Agent Terminology
The following terms are more frequently used in reinforcement learning in AI −
- Performance Measure of Agent − It is the criteria, which determines how successful an agent is.
- Behavior of Agent − It is the action that agent performs after any given sequence of percepts.
- Percept − It is agent’s perceptual inputs at a given instance.
- Percept Sequence − It is the history of all that an agent has perceived till date.
- Agent Function − It is a map from the precept sequence to an action.
Environment
- Some programs operate in an entirely artificial environment confined to keyboard input, database, computer file systems and character output on a screen.
- In contrast, some software agents, such as software robots or softbots, exist in rich and unlimited softbot domains. The simulator has a very detailed, and complex environment. The software agent needs to choose from a long array of actions in real time.
- For example, a softbot designed to scan the online preferences of the customer and display interesting items to the customer works in the real as well as an artificial environment.
Properties of Environment
The environment has multifold properties as discussed below −
- Discrete/Continuous − If there are a limited number of distinct, clearly defined, states of the environment, the environment is discrete , otherwise it is continuous. For example, chess is a discrete environment and driving is a continuous environment.
- Observable/Partially Observable − If it is possible to determine the complete state of the environment at each time point from the percepts, it is observable; otherwise it is only partially observable.
- Static/Dynamic − If the environment does not change while an agent is acting, then it is static; otherwise it is dynamic.
- Single agent/Multiple agents − The environment may contain other agents which may be of the same or different kind as that of the agent.
- Accessible/Inaccessible − If the agent’s sensory apparatus can have acc
ess to the complete state of the environment, then the environment is accessible to that agent; otherwise it is inaccessible.
- Deterministic/Non-deterministic − If the next state of the environment is completely determined by the current state and the actions of the agent, then the environment is deterministic; otherwise it is non-deterministic.
- Episodic/Non-episodic − In an episodic environment, each episode consists of the agent perceiving and then acting. The quality of its action depends just on the episode itself. Subsequent episodes do not depend on the actions in the previous episodes. Episodic environments are much simpler because the agent does not need to think ahead.
Constructing an Environment with Python
For building reinforcement learning agent, we will be using the OpenAI Gym package which can be installed with the help of the following command −
pip install gym
There are various environments in OpenAI gym which can be used for various purposes. Few of them are Cartpole-v0, Hopper-v1, and MsPacman-v0. They require different engines. The detail documentation of OpenAI Gym can be found on https://gym.openai.com/docs/#environments.
The following code shows an example of Python code for cartpole-v0 environment −
import gym
env = gym.make('CartPole-v0')
env.reset()
for _ in range(1000):
env.render()
env.step(env.action_space.sample())
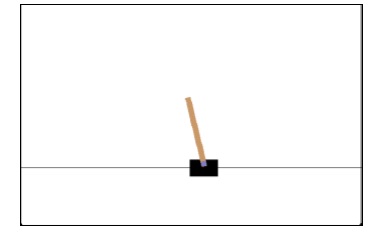
You can construct other environments in a similar way.
Constructing a learning agent with Python
For building reinforcement learning agent, we will be using the OpenAI Gym package as shown −
import gym
env = gym.make('CartPole-v0')
for _ in range(20):
observation = env.reset()
for i in range(100):
env.render()
print(observation)
action = env.action_space.sample()
observation, reward, done, info = env.step(action)
if done:
print("Episode finished after {} timesteps".format(i+1))
break
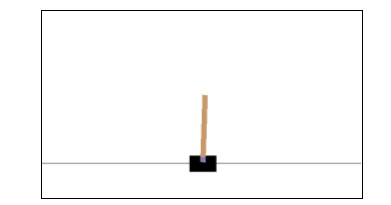
Observe that the cartpole can balance itself.
[wpsbx_html_block id=1891]
by Jesmin Akther | Aug 30, 2021 | Artificial Intelligence
Artificial Intelligence research areas and Agent are discussed in the article. Here firstly we are going to know about speech and voice recognition.
Speech and Voice Recognition
These both terms are common in robotics, expert systems and natural language processing. Though these terms are used interchangeably, their objectives are different.
Speech Recognition |
Voice Recognition |
The speech recognition aims at understanding and comprehending WHAT was spoken. |
The objective of voice recognition is to recognize WHO is speaking. |
It is used in hand-free computing, map, or menu navigation. |
It is used to identify a person by analyzing its tone, voice pitch, and accent, etc. |
Machine does not need training for Speech Recognition as it is not speaker dependent. |
This recognition system needs training as it is person oriented. |
Speaker independent Speech Recognition systems are difficult to develop. |
Speaker dependent Speech Recognition systems are comparatively easy to develop. |
Working of (Speech and Voice) Recognition Systems
The user input spoken at a microphone goes to sound card of the system. The converter turns the analog signal into equivalent digital signal for the speech processing. The database is used to compare the sound patterns to recognize the words. Finally, a reverse feedback is given to the database. This source-language text becomes input to the Translation Engine, which converts it to the target language text. They are supported with interactive GUI, large database of vocabulary, etc.
Real Life Applications of Research Areas
There is a large array of applications where AI is serving common people in their day-to-day lives:
Expert Systems
Examples − Flight-tracking systems, Clinical systems.
Natural Language Processing
Examples: Google Now feature, speech recognition, Automatic voice output.
Neural Networks
Examples − Pattern recognition systems such as face recognition, character recognition, handwriting recognition.
Robotics
Examples − Industrial robots for moving, spraying, painting, precision checking, drilling, cleaning, coating, carving, etc.
Fuzzy Logic Systems
Examples − Consumer electronics, automobiles, microwave oven etc.
The domain of AI is classified into Formal tasks, Mundane tasks, and Expert tasks.
Task Domains of Artificial Intelligence |
Ordinary Tasks |
Formal Tasks |
Expert Tasks |
Perception
- Computer Vision
- Speech, Voice
|
- Mathematics
- Geometry
- Logic
- Integration and Differentiation
|
- Engineering
- Fault Finding
- Manufacturing
- Monitoring
|
Natural Language Processing
- Understanding
- Language Generation
- Language Translation
|
Games
- Go
- Chess (Deep Blue)
- Ckeckers
|
Scientific Analysis |
Common Sense |
Verification |
Financial Analysis |
Reasoning |
Theorem Proving |
Medical Diagnosis |
Planing |
|
Creativity |
Robotics
|
|
|
[wpsbx_html_block id=1891]
Humans learn ordinary (mundane ) tasks since their birth. They learn by perception, speaking, using language, and locomotives. They learn Formal Tasks and Expert Tasks later, in that order. For humans, the mundane tasks are easiest to learn. The same was considered true before trying to implement mundane tasks in machines. Earlier, all work of AI was concentrated in the mundane task domain. Later, it turned out that the machine requires more knowledge, complex knowledge representation, and complicated algorithms for handling mundane tasks. This is the reason why AI work is more prospering in the Expert Tasks domain now, as the expert task domain needs expert knowledge without common sense, which can be easier to represent and handle.
Agent and Environment:
An agent is anything that can perceive its environment through sensors and acts upon that environment through effectors.
- A human agent has sensory organs such as eyes, ears, nose, tongue and skin parallel to the sensors, and other organs such as hands, legs, mouth, for effectors.
- A robotic agent replaces cameras and infrared range finders for the sensors, and various motors and actuators for effectors.
- A software agent has encoded bit strings as its programs and actions.
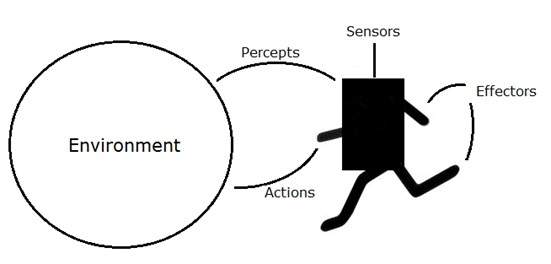
Agent Terminology
- Performance Measure of Agent: It is the criteria, which determines how successful an agent is.
- Behavior of Agent: It is the action that agent performs after any given sequence of percepts.
- Percept: It is agent’s perceptual inputs at a given instance.
- Percept Sequence: It is the history of all that an agent has perceived till date.
- Agent Function: It is a map from the precept sequence to an action.
Rationality
Rationality is a status of being reasonable, sensible, and having good sense of judgment. It is concerned with expected actions and results depending upon what the agent has perceived. Performing actions with the aim of obtaining useful information is an important part of rationality.
Ideal Rational Agent:
An ideal rational agent is the one, which is capable of doing expected actions to maximize its performance measure, on the basis of −
- Its percept sequence
- Its built-in knowledge base
Rationality of an agent depends on the following −
- The performance measures, which determine the degree of success.
- Agent’s Percept Sequence till now.
- The agent’s prior knowledge about the environment.
- The actions that the agent can carry out.
A rational agent always performs right action, where the right action means the action that causes the agent to be most successful in the given percept sequence. The problem the agent solves is characterized by Performance Measure, Environment, Actuators, and Sensors (PEAS).
The Structure of Intelligent Agents
Agent’s structure can be viewed as:
- Agent = Architecture + Agent Program
- Architecture = the machinery that an agent executes on.
- Agent Program = an implementation of an agent function.
Simple Reflex Agents
- They choose actions only based on the current percept.
- They are rational only if a correct decision is made only on the basis of current precept.
- Their environment is completely observable.
Condition-Action Rule − It is a rule that maps a state (condition) to an action.
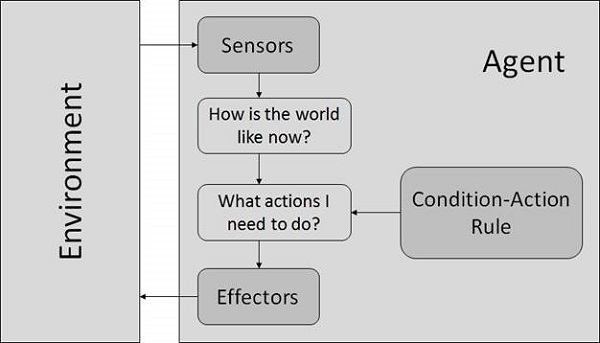
Model Based Reflex Agents
They use a model of the world to choose their actions. They maintain an internal state.
Model − knowledge about “how the things happen in the world”.
Internal State − It is a representation of unobserved aspects of current state depending on percept history.
Updating the state requires the information about:
- How the world evolves.
- How the agent’s actions affect the world.

Goal Based Agents
They choose their actions in order to achieve goals. Goal-based approach is more flexible than reflex agent since the knowledge supporting a decision is explicitly modeled, thereby allowing for modifications.
Goal − It is the description of desirable situations.

Utility Based Agents
They choose actions based on a preference (utility) for each state.
Goals are inadequate when;
- There are conflicting goals, out of which only few can be achieved.
- Goals have some uncertainty of being achieved and you need to weigh likelihood of success against the importance of a goal.
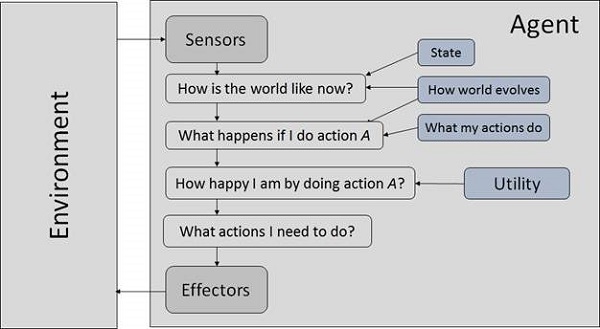
The Nature of Environments
Some programs operate in the entirely artificial environment confined to keyboard input, database, computer file systems and character output on a screen. Besides, some software agents (software robots or softbots) exist in rich, unlimited softbots domains. The simulator has a very detailed, complex environment. The software agent needs to choose from a long array of actions in real time. A softbot designed to scan the online preferences of the customer and show interesting items to the customer works in the real as well as an artificial environment. The most famous artificial environment is the Turing Test environment, in which one real and other artificial agents are tested on equal ground. This is a very challenging environment as it is highly difficult for a software agent to perform as well as a human.
Turing Test: The success of an intelligent behavior of a system can be measured with Turing Test.
Two persons and a machine to be evaluated participate in the test. Out of the two persons, one plays the role of the tester. Each of them sits in different rooms. The tester is unaware of who is machine and who is a human. He interrogates the questions by typing and sending them to both intelligences, to which he receives typed responses. This test aims at fooling the tester. If the tester fails to determine machine’s response from the human response, then the machine is said to be intelligent.
Properties of Environment
The environment has multifold properties: Discrete / Continuous − If there are a limited number of distinct, clearly defined, states of the environment, the environment is discrete (For example, chess); otherwise it is continuous (For example, driving).
- Observable / Partially Observable: If it is possible to determine the complete state of the environment at each time point from the percepts it is observable; otherwise it is only partially observable.
- Static / Dynamic: If the environment does not change while an agent is acting, then it is static; otherwise it is dynamic.
- Single agent / Multiple agents: The environment may contain other agents which may be of the same or different kind as that of the agent.
- Accessible / Inaccessible: If the agent’s sensory apparatus can have access to the complete state of the environment, then the environment is accessible to that agent.
- Deterministic / Non-deterministic: If the next state of the environment is completely determined by the current state and the actions of the agent, then the environment is deterministic; otherwise it is non-deterministic.
- Episodic / Non-episodic: In an episodic environment, each episode consists of the agent perceiving and then acting. The quality of its action depends just on the episode itself. Subsequent episodes do not depend on the actions in the previous episodes. Episodic environments are much simpler because the agent does not need to think ahead.